The evolution of novel data processing technologies is fast paced and the volume of data being generated is growing by the second. The food industry stands to benefit from this and has been testing and adapting various routes for using data science techniques to enhance the production of safe and healthy foods.
Data science requires a multidisciplinary approach and a broad range of skill sets, from mathematics and statistics, computer science and machine learning to artificial intelligence (AI). Data science also needs to have strong ties to the actual domain knowledge (1) in order to ask the right questions and select the right data. Predictive analytics and scientific modelling are interesting areas of data science and the activity in this space is growing. Applications can range from traditional methods using advanced statistics for assessing various future scenarios to machine learning techniques, including artificial intelligence.
The use of data science within food and health has become more prevalent and has been steadily complementing more traditional approaches. Predictive modelling for making informed decisions in new product development, business strategy and consumer health and safety has now demonstrated its value to stakeholders from industry, governments and research organisations on many occasions, some of which are described below.
The collecting, centralising and formatting of data via spreadsheets, hardcopies, documents, IOT or other means is the first step into digitisation of data, followed by the structuring, validating, analysing and visualising of this data. Only then is it possible to develop more advanced models that serve to inform R&D (from product design to launch), safety (including exposure and microbial food safety), consumer health and strategy.
Probabilistic Exposure Modelling
Probabilistic Exposure Modelling has been used and applied for a number of decades. As part of an overall risk assessment of a food contaminant, pesticide residue, additive or a novel ingredient on a population of consumers, an exposure assessment has to be carried out. As an approximation, the exposure can be quantified by the amount of food consumed multiplied by the concentration of the contaminant in this food. However, when looking at exposure within and across consumer populations, this simple calculation can become quite complex. A chemical can be present at varying levels in a large variety of foods, consumed in varying quantities, in different combinations, by different consumers in different countries/regions, and at different life stages.
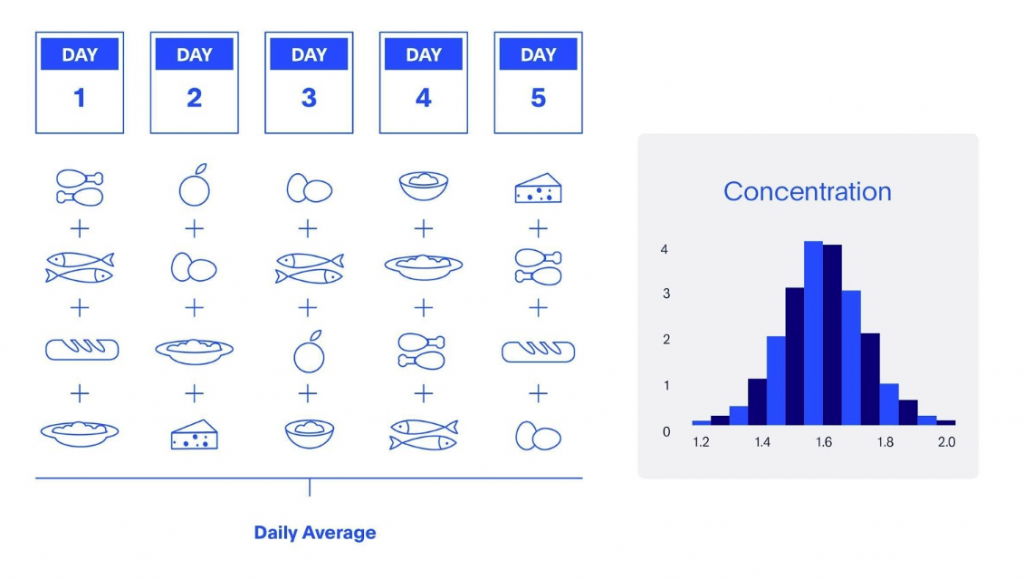
Therefore, exposure in a population is intrinsically variable and has a number of sources of uncertainty; this variability and uncertainty should be captured using probabilistic methods in each risk assessment scenario, as required. The results can then be expressed with confidence bounds and the scenarios can be evaluated more rigorously.
As an initial screening exercise, more simplistic methods are often applied to estimate exposure, high level consumption statistics and average or maximum chemical concentration levels. When comparing those crude exposure levels to health based thresholds, such as the acceptable daily intake (ADI) or tolerable daily intake (TDI), this can become an issue as the exposure is likely to be overestimated and will potentially exceed those limits, especially when exposure is aggregated from multiple sources.
This is where probabilistic dietary exposure modelling comes into its own to refine the exposure results for a population in a far more accurate and realistic manner by applying various mathematical techniques, such as using distributions of intakes, accounting for a range of concentrations rather than using a mean or a maximum point value, occurrence of a chemical within a food, and so on. Probabilistic data can be represented by parametric or empirical distributions, integrated in the analysis using Monte Carlo simulations.
New product development and its impact on nutrition and health
Another example of using data science, and specifically predictive models, in the food industry is to assess the impact of a dietary change on nutritional intakes and subsequently health outcomes. This change can consist of a new product formulation, a new food or ingredient, a reformulated product or a portion size change. The impact of this dietary change on consumers can be assessed by using nutritional intake modelling. As with exposure modelling, data on food consumption is required.
Food consumption surveys assess population dietary behaviour and health at national and local level in various geographies using specific survey methodologies. If available, individual consumption data can be used to model the impact of dietary changes on intakes. These food consumption databases vary in quality, size and detail but usually report information at eating event level for each representative participant within the survey. Food descriptions, consumed amounts and a diary of consumption events are recorded as well as nutrient composition data for each food. The number of consumers representing a given population can range from a couple of hundred to tens of thousands; the number of consumers surveyed is chosen to be large enough to be statistically representative of the population. The individual foods recorded as consumed can range from 500 to up to 10,000 foods, usually categorised into specific food groups. Having access to such granular databases enables very targeted analysis.
One such study (2) investigated the impact of a new milk powder in China, a country where the burden of cardiovascular disease is on the incline. Potassium has been shown to reduce systolic blood pressure in pre-hypertensive consumers. Using a scientific model, a milk powder fortified with potassium was introduced into the Chinese diet.
The underlying data used in this model consisted of individual eating event level data on food consumption and composition of foods, representing the Chinese population (China Health and Nutrition Survey – CHNS) as well as the composition data of the new milk product. The composition data used for the foods consumed in the CHNS was obtained from the Institute of Nutrition and Food Safety, China CDC (2004) and Institute of Nutrition and Food Safety, China CDC (2002). The survey includes information for 21 food groups and 1,599 foods. Anthropometric measurements, blood pressure and biomarker data were also collected in this survey. The target age group was 45 years and older, which resulted in 6,134 subjects, whose dietary intakes were monitored.
Within this age group the new milk powder was either substituted for normal milk or added on top of the normal diet via different scenarios and depending on the consumers’ potassium intakes. Potassium intake distributions were assessed at baseline and after substitution. Individual increases in intake were calculated as well as the overall shift in population intakes.
Based on findings from the literature, the increase of potassium intakes and the resulting decrease in systolic blood pressure were assessed. Individual consumers’ blood pressure within the survey data was then modified to account for the impact on blood pressure resulting from the milk substitution.
The benefit to a food company of an analysis such as the above is to assess whether a product will provide a health benefit for a targeted consumer population and to quantify the possible impact.
1 – Data Science Field/.Term Diagram: Ryan Urbanowicz, PhD University of Pennsylvania, Philadelphia PA, 19104
2 – Dainelli L, Xu T, Li M, Zimmermann D, Fang H, Wu Y, Detzel P. 2017. Cost-effectiveness of milk powder fortified with potassium to decrease blood pressure and prevent cardiovascular events among the adult population in China: a Markov model. BMJ Open [Internet]. 7:e017136. Available from: http://bmjopen.bmj.com/lookup/doi/10.1136/bmjopen-2017-017136